What is an unsupervised classification?
¿Qué es la clasificación no supervisada?
From an engineering perspective, the simplest use of optical remotely sensed imagery is on the interpretation and identification of historical changes of land surface features (e.g., on the area covered by a certain kind of forest). Using simple words, such task can be accomplished by classifying the pixels of the imagery into as many groups as needed, or more properly, as allowed by the resolution of the imagery. There are two kinds of classification procedures: the unsupervised classification and the supervised classification. The supervised classification is principally used to extract qualitative information out from the imagery, having enough knowledge of the physical characteristics of some pixels, which can be used to characterize or classify other pixels within the study area. On the other hand, the unsupervised classification, simply generates clusters of pixels, that qualitatively characterize the study area. The latter is commonly used when there is not enough knowledge on the physical characteristics of the pixels (e.g., when performing multi temporal analysis of surface areas along a wide historical lapse of time, for which there is limited knowledge and/or information). An interesting example can be found following the link of the figure above. In this entry, I am presenting a tutorial of how to perform the latter (unsupervised classification). The material has been originally prepared by the consultants C. Buitrón and J. Fernández, under my supervision. This tutorial follows the series published on the entries presented before on this blog. Enjoy!
- Open the image, e.g., l519971016_stack_cut_corr_hz. On the tools bar go to DRAWING →InsertGeometry→ polygon or rectangle and delimítate the area of interest. Then, go to FILE → Save as →AOI layer as save (AOI_1) and close.
- Go to RASTER → CLASSIFICATION → Unsupervised→ Unsupervised Classification, load the image in “Input raster file”. On “Output clusterlayer” create the folder UNSUPERVISED CLASSIFICATION and save the image as, e.g., l519971016_stack_cut_corrected_hz_clas_no_sup.. On “Output Signature Set” create a folder SIGNATURES and save it under the name of the image (e.g., l519971016).
- On “Number of classes” write 40 and on “Max Iterations” write 20 (the number of iterations has been found following an empirical iterative procedure, because of which it may vary according to the feature studied).
- Go to “Initializing Options” and select “Principal Axis” and close it,
- Go to “Color Scheme Options”→“Greyscale”→ Close.
- Go to “AOI”→ AOI file and open the AOI _1 previously created (this AOI can be used for the remaining images, if the study area and the AOI are the same), finally OK.
- On the tools bar, go to HOME →Add Views→Create New 2D View.
- To the left it will appear 2D View #2; open the image: l519971016_stack_cut_corrected_hz_clas_no_sup, (you will see that the image appears in grey tones).
- Go to link views→ link views for both images: l519971016_stack_cut_corrected_hz (Go to MULTIESPECTRAL and set up the combination layer 5, layer 4 and layer 3, in order to highlight the snow cover), and the image l519971016_stack_cut_corrected_hz_clas_no_sup. Now you will realice that both images move together, at the same time.
- With the image activated, e.g., l519971016_stack_cut_corrected_hz_clas_no_sup, go to the tool bar on TABLE → SHOW ATRIBUTES and a table on the lower part will come up (attributes table). On the selected image, a certain number of rows will represent the desired feature (e.g., snow). Now we should confirm whether or not the desired feature is well represented.
- In order to better visualize and classify the desired feature, on the table, go to ROW and click on the number. On the “COLOR” column, click on the right bottom of the mouse and paint the color of the feature we wish to select (e.g., yellow). Now you will realize that all the values represented by a given color will change, highlighting in that way the feature we area interested in.
- Once the desired feature is painted, we close and save changes.
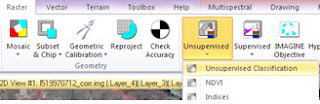
No comments:
Post a Comment